In the days before World War I a physicist by the name of Emanuel Goldberg created a machine that could read characters and convert them into telegraph code; it was the beginning of what we now know as Optical Character Recognition (OCR). Things have moved on since then. Since the 1970s, OCR has been able to read text in a wide variety of fonts, in the 1980s it moved onto office computers, then in the early 2000s, OCR was made available as a service via the internet.
For some 20 years now traditional players in the market have been looking for ways to transform their OCR output into a usable data format for businesses. In 90% of instances, this required either keyword/key value matching or hardcoded templates to find relevant text.
In recent years, AI has transformed the field, far surpassing solutions that don’t use AI in terms of performance and maintenance and rendering them largely obsolete.
Sunk Costs and Familiarity
Many businesses are hesitant at making the jump to IDP and are still throwing money at OCR.
There is a growing consensus that OCR is not an effective answer to the surging tide of document-based data that many businesses are trying to navigate. But OCR is being maintained due to sunk costs and familiarity.
But legacy software brings with it other costs, many of them less immediately obvious than the extra work that stems from poor document-processing accuracy:
There’s a cost in terms of the employee experience. Having to struggle with the clunky, out-of-date design of legacy software is frustrating.
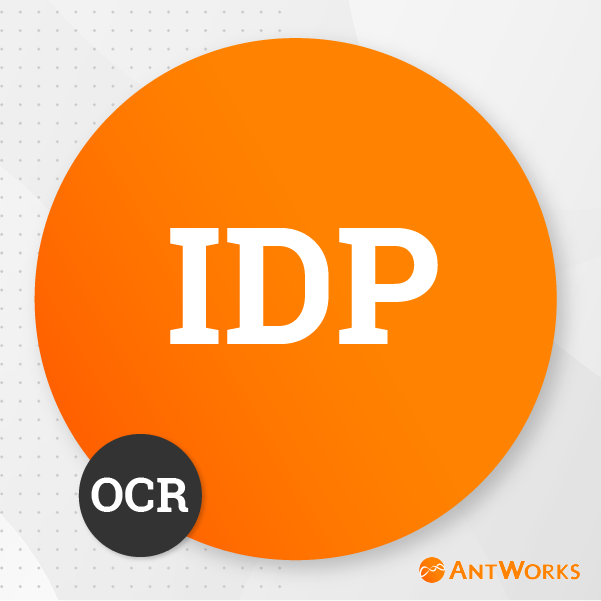
Many of the older OCR engines have been in use for over 20 years, and their tangled web of complex modules/pop-ups often goes all the way back to the Windows 98 era. Most people positively like to feel productive and want something to show for their efforts. Inefficient OCR systems make their users look and feel like they’re not achieving much.
Intelligent document processing software as a service (SaaS) is constantly being updated to incorporate cutting-edge technology that vastly improves the user experience.
A business’s own programmers and system administrators also have to deal with legacy systems, generally at a far deeper level. You can multiply the feelings of frustration that end users experience. According to Evans Data Corp, the cost of bad code to the global economy is as high as $85.0 billion per year. Spending money to support outdated software might feel like the safe and familiar option but it’s like keeping an old car on the road minus the romance.
If that hasn’t persuaded you, in this blog I’ll lay out two further reasons why you should dump outmoded OCR and move to AI-driven IDP.
Feedback Loop
Conventional document processing solutions lack the capacity to improve through user feedback. Typically, businesses that rely on non-AI systems either have to spend a lot of time and money on having software company consultants create and maintain a set of templates (for documents from each vendor, customer, partner, etc. they work with) or spend a lot of time and money doing it themselves. Without this labour-intensive work whenever the format of a documents you receive changes your automation will stop working properly.
In contrast, an IDP platform has three distinct features that set it apart:
- It eliminates the need to create and maintain separate templates for each client or vendor because it searches for data in sophisticated ways that emulate the ways people search for data. Whereas OCR is limited to extracting specific data from specific locations in documents that it’s been trained to process, IDP can find data anywhere in a document.
- Verification and validation creates a “Feedback-Loop” that allows the platform to learn and improve over time with the aid of Machine Learning models.
- The model has in-built “Roll-back” mechanisms that allow it to use older versions of itself.
IDP is designed around self-improvement and makes it simple for users to provide feedback that is incorporated into the model’s iterative improvement process. Traditional OCR providers sometimes claim to make use of AI for marketing reasons, but unless a system is built bottom-up from AI components it won’t have the capacity to learn and improve by itself, nor process data in the human-like ways that are the hallmark of IDP.
Improved Accuracy
The accuracy of a document processing or automation project can be evaluated at various stages and through a variety of metrics. Now, here’s something we need to be frank about; the initial step of the IDP process does use OCR. As a technology it’s still useful for converting an image into text. This is the first point at which accuracy can be measured; here by the percentage of input characters (letters, numbers, etc.) that were successfully read and converted. In the past, for instance, poor OCR engines could not tell the difference between the digits one and seven, or between the letter o and the digit zero.
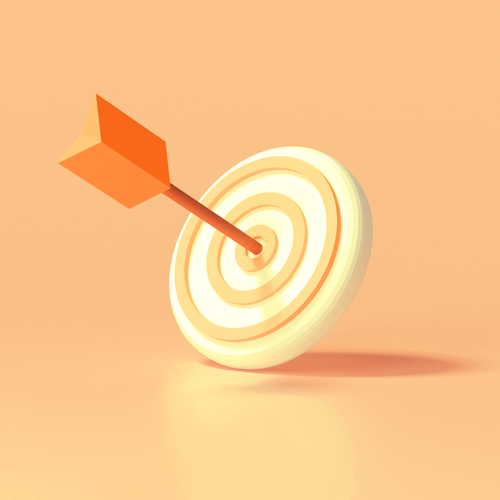
These days, however, OCR technology has improved to the point where such errors are increasingly rare, though it certainly struggles with handwritten text in cursive and other low-contrast formats, as can IDP. With IDP however, there is a higher level of confidence for data extraction even when it comes to cursive handwriting.
Adopting an IDP platform will not just increase your document data extraction accuracy but, over time, you’ll see that accuracy constantly edging closer to 100%. OCR will simply fall further and further behind.
Setting the Stage for IDP
Accessing analogue data can be difficult, especially in large, geographically dispersed organisations where it can be located hundreds or thousands of miles away. But with IDP, it can be converted into digital, machine-readable data through a process that includes classification, extraction, and validation.
Classification – Documents submitted to the IDP are automatically categorised using machine learning.
Extraction – Whether images are handwritten, machine printed, or poorly scanned, the system uses computer vision to extract the relevant fields.
Validation – The system can assess its confidence in the accuracy of the extraction and, if necessary, can mark the output for human inspection.
Keeping humans in the loop is a critical aspect of IDP. Staff monitor IDP functionality, investigate reported issues, and contribute to the system’s ongoing development.
The IDP ROI Explained
Intelligent self-learning has the potential to transform IDP accuracy and, in the long run, reduce the need for human intervention.
Reducing the amount of time colleagues spend on repetitive, manual document reading tasks cuts errors and the time saved either allows businesses to reduce head count or to redeploy skilled people to tasks that grow the business. IDP can help by providing extracted data in a standard output thereby helping companies save time and effort in training and re-training employees.
Lower costs mean higher profits and an ROI within a far tighter time frame than is usual with technology implementations. The number of channels used and the number of process journeys that receive analogue data determine how quickly margins can improve.
In addition to its flexibility, IDP’s scalability means it can be used by businesses of all sizes and in a variety of contexts. As capabilities increase, scaling across the organisation becomes less of a challenge, and centralisation and the development of enterprise-wide utilities present new possibilities. By fostering cooperation between humans and machines, companies can boost output.
The IDP Verdict
Analogue information locked away in paper documents, be it structured, semi-structured, or unstructured, is too valuable to be wasted. Being able to find hidden patterns in data that were previously ignored leads to faster, better-informed decision making and that confers a strategic advantage. There are many obstacles to digitising data and extracting value from it, but, implemented properly, IDP can help with both. Businesses can improve their productivity and precision with the help of their digitised data in the here-and-now, while also gaining insights and unlocking capacity to boost enterprise effectiveness and productivity in the future.